연구
HOME > 연구 > 연구성과
연구성과
자율 주행 기술 개발
VDC Lab 자율주행 시연 영상 (KAIST 문지캠퍼스)
SLAM (Simultaneous Localization And Mapping)
- GPS등 위치 인식 센서는 차량의 위치 인식정보를 제공하지만 도시환경에서는 빌딩으로부터의 간섭 때문에 lane-level accuracy를 보장하지 못하고 이는 자율주행에 있어서 문제가 될 수 있음
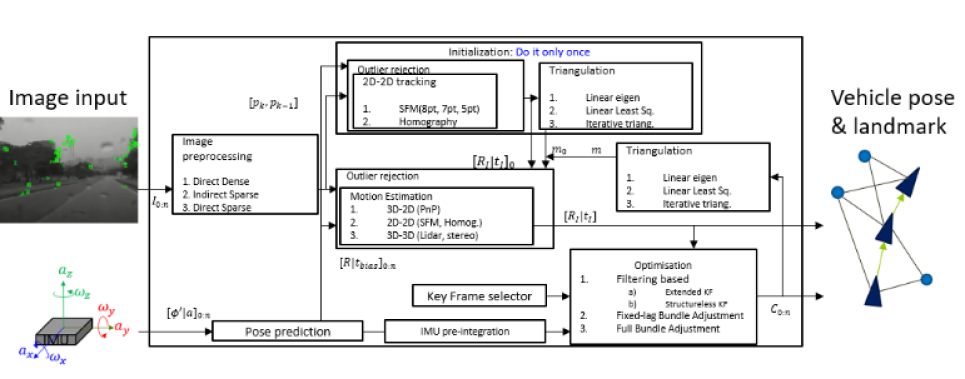
- 카메라 관성계를 이용하여 센서를 융합하여 gps같은 절대적 위치 좌표가 없는 경우에도 랜드마크 지도와 자기 자신을 검출하는 SLAM 프레임워크 개발
딥러닝 기반 3차원 객체 검출 기술
- 3차원 객체 검출 기술은 자율주행 차량 주변의 동적/정적 객체의 위치, 크기를 검출하는 기술로, 검출된 객체의 정보는 자율주행 차량의 미래 경로를 계획하는데 사용되므로 매우 중요함.
- 자율주행 기술저비용의 카메라, 레이다 센서를 게이팅 메커니즘을 이용하여 센서 융합하여 강인하게 객체를 검출히는 딥러닝 네트워크 기술 개발을 개발하는데 있어서 차량 움직임 예측 기술은 자율주행 차량의 경로를 계획하는데 필수적인 기술

GRIF Net (IROS 2020)
관련 논문
- Kim et al., “Deep Learning based Vehicle Position and Orientation Estimation via Inverse Perspective Mapping Image”, IEEE Intelligent Vehicles Symposium (IV), 2020
- Kim et al., “GRIF Net : Gated Region of Interest Fusion Network for Robust 3D Object Detection from Radar Point Cloud and Monocular Image”, IEEE/RSJ International Conference on Intelligent Robots and Systems (IROS), 2020
- Kim et al., “Low-level Sensor Fusion for 3D Vehicle Detection using Radar Range-Azimuth Heatmap and Monocular Image”, AFCV Asian Conference on Computer Vision (ACCV), 2020
딥러닝 기반 차량 움직임 예측
- 자율주행 기술을 개발하는데 있어서 차량 움직임 예측 기술은 자율주행 차량의 경로를 계획하는데 필수적인 기술
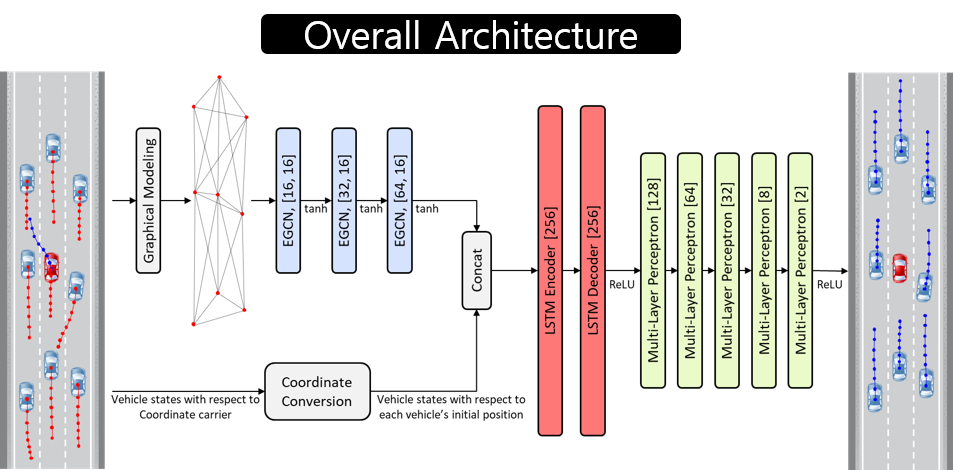
SCALE-Net 의 전체 구조
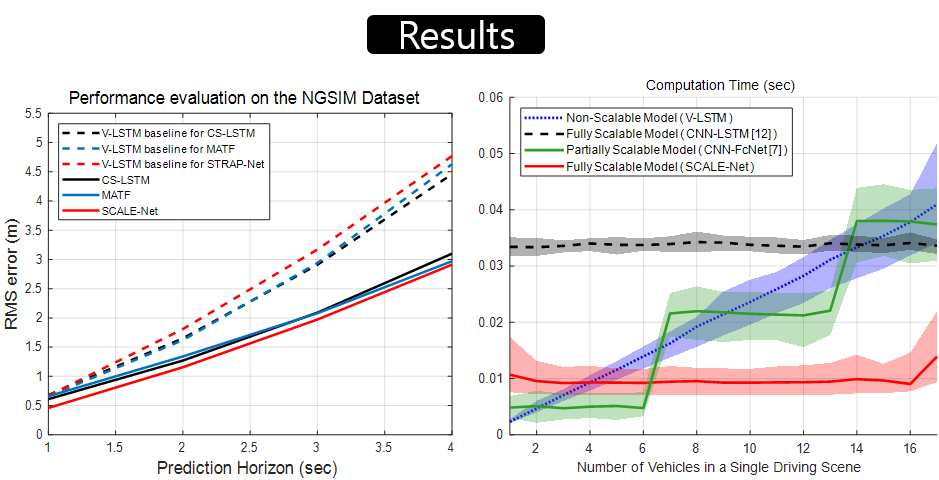
SCALE-Net을 이용한 주변차량 예측 결과
SCALE-Net (IROS 2020)
- 주변 차량의 움직임을 정확하게 예측하기 위해서 다양한 딥러닝 기술(EGCN, LSTM 등)을 활용한 예측 기술을 개발
- 정확한 미래 위치를 예측하는 예측의 정확성뿐만 아니라 자율주행 차량 주변에 존재하는 차량 대수의 변화에 강인한 예측 기술을 개발
관련 논문
- Jeon et al., “Traffic Scene Prediction via Deep Learning: Introduction of Multi-Channel Occupancy Grid Map as a Scene Representation”, IEEE Intelligent Vehicles Symposium (IV), 2018
- Yoon et al., “Predictive Cruise Control using Radial Basis Function Network-based Vehicle Motion Prediction and Chance Constrained Model Predictive Control”, IEEE Transactions on Intelligent Transportation Systems, 2019
- Kim et al., “RECUP Net: RECUrsive Prediction Network for Surrounding Vehicle Trajectory Prediction with Future Trajectory Feedback”, IEEE International Conference on Intelligent Transportation Systems (ITSC), 2020
- Jeon et al., “SCALE-Net: Scalable Vehicle Trajectory Prediction Network under Random Number of Interacting Vehicles via Edge-enhanced Graph Convolutional Neural Network” IEEE/RSJ International Conference on Intelligent Robots and Systems (IROS), 2020
강화학습 기반 차량 경로 생성 기술
- 강화학습을 활용한 자율주행 차량의 안전한 주행 경로 생성 기술 개발
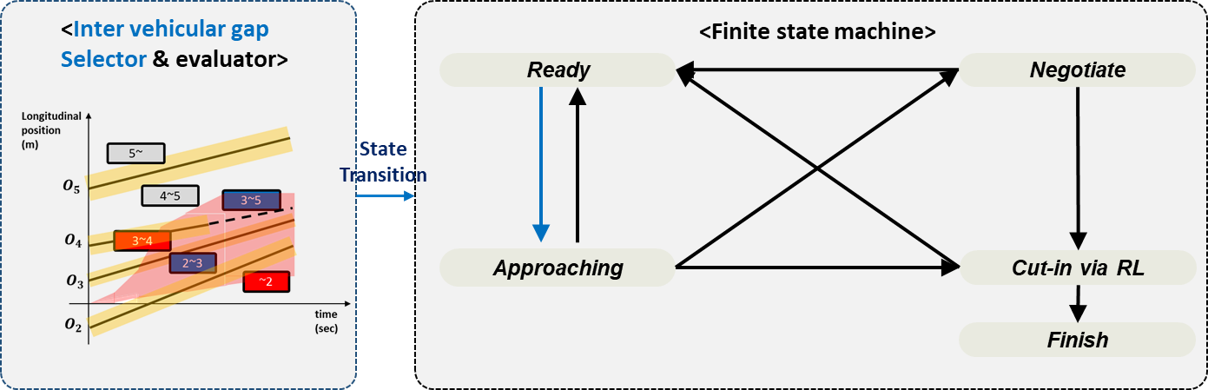
유한 요소 기계 기반 판단 기술
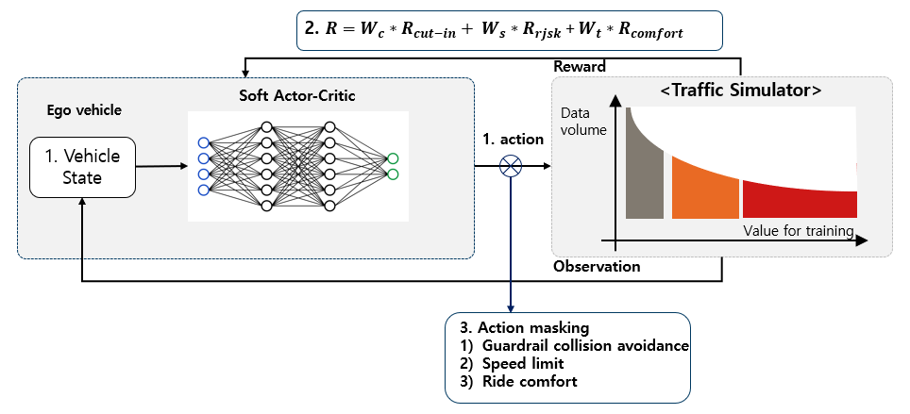
정책 기반 강화학습을 이용한 경로 생성
- 유한 요소 기계를 활용한 High-Level 의사결정을 통한 목표 주행 상태 결정 후 Soft Actor-Critic 기반 강화학습을 활용한 차선 변경 경로 생성
- Cut-in 성공률 및 충돌회피율 향상 (level 6 에 해당하는 복잡한 교통 상황에서 충돌 회피율 99.92% 및 Cut-in 성공률 77.18%)
전기동력시스템 개발
HEV 아키텍처 개발
- Power-split HEV는 매우 효율적이며 고성능의 EV 모드가 가능한 HEV로 full 하이브리드 와 Plug-in 하이브리드에 모두 적합

The comprehensive design process of power-split hybrid electric vehicles
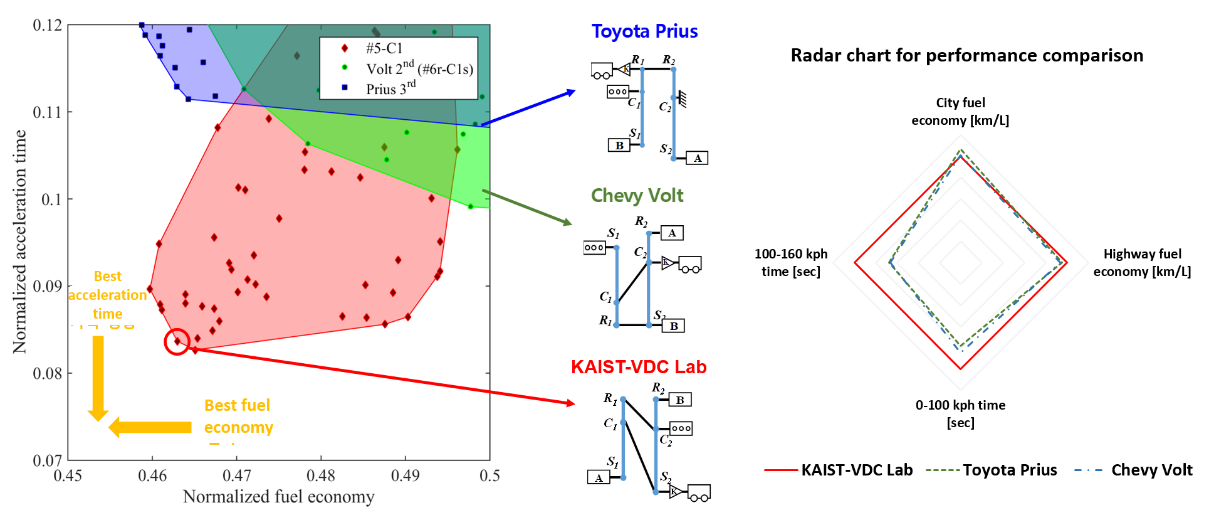
Performance Assessment Map (designs toward left bottom side have outstanding performance)
- 가상 설계 공간을 이용한 최적의 Power-split하이브리드 파워트레인 아키텍처를 찾기 위한 혁신적인 방법 고안
- 세계적 수준의 연비와 가속 성능을 보유한 새로운 Power-split 하이브리드 아키텍처 제시 (도요타 Prius 대비 27.8%, 쉐보레 Volt 대비 26.2% 빠르고, 3.3% 효율적)
관련 논문
- Kang et al., “Systematic Design of Input-and Output-Split Hybrid Electric Vehicles With a Speed Reduction/Multiplication Gear Using Simplified-Lever Model”, IEEE Transactions on Intelligent Transportation Systems, 2019
- Kim et al., “Comprehensive Design Methodology of Compound-Split Hybrid Electric Vehicles: Introduction of the Compound Lever as a Design Tool” IEEE Access, 2019
- Barhoumi et al,. “Automatic generation of design space conversion maps and its application for the design of compound split hybrid powertrains” Journal of Mechanical Design, 2018
- Barhoumi & Kum, “Automatic enumeration of feasible kinematic diagrams for split hybrid configurations with a single planetary gear” Journal of Mechanical Design, 2017
- Kim & Kum. “Comprehensive design methodology of input-and output-split hybrid electric vehicles: In search of optimal configuration” IEEE/ASME Transactions on Mechatronics, 2016
- Barhoumi et al., “Compound lever based optimal configuration selection of compound-split hybrid vehicles” SAE Technical Paper, 2017
- Kim et al., “Impact of speed reduction (multiplication) gear on the performance of input-and output-split hybrid electric vehicles” SAE Technical Paper, 2017
도그클러치 모델 개발
- 효율이 낮은 마찰 클러치 대신 유압 시스템이 필요하지 않아 구조가 간단하며 마찰이 아닌 결합을 통해 동력을 전달하여 동력 전달 효율이 높은 도그 클러치 기술 개발
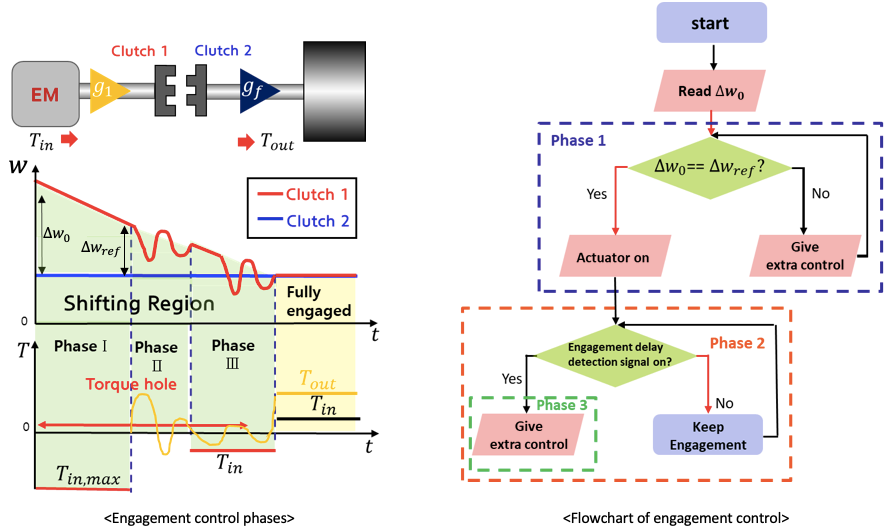
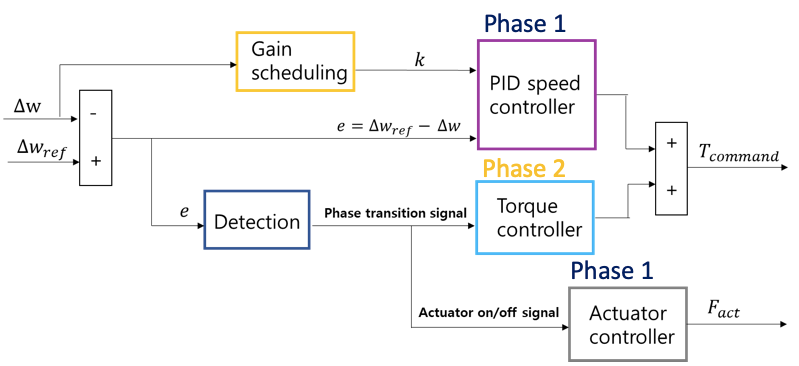
Dog clutch engagement controller
- 도그 클러치 체결 충격과 시간에 가장 중요한 요소인 치형 사이의 접촉력뿐만 아니라 테이퍼 각도, 백래시, 치형 등 다양한 설계 변수를 고려한 도그 클러치 모델 개발.
- 상대 속도를 체결 가능한 속도 범위에 맞춰 체결 지연을 방지하는 제어 알고리즘을 개발을 통한 체결 완료 시간 최대 60%까지 향상
Engine start control for parallel HEVs
- Pre-transmission parallel HEV의 경우 엔진을 크랭킹하기 위한 starter motor의 사이즈와 종류에 따라 다양한 아키텍쳐가 존재
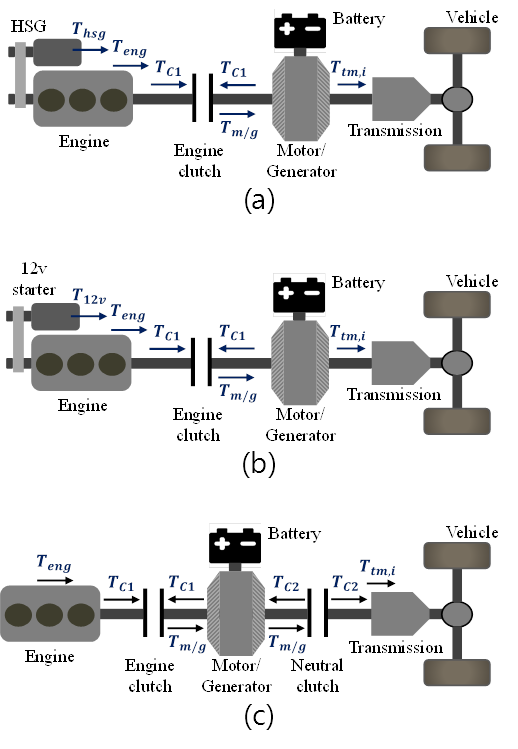
Structure diagram of the pre-transmission parallel HEV with various starter motors. (a) P0-P2 type. (b) 12v+P2 type. (c) P2 only type
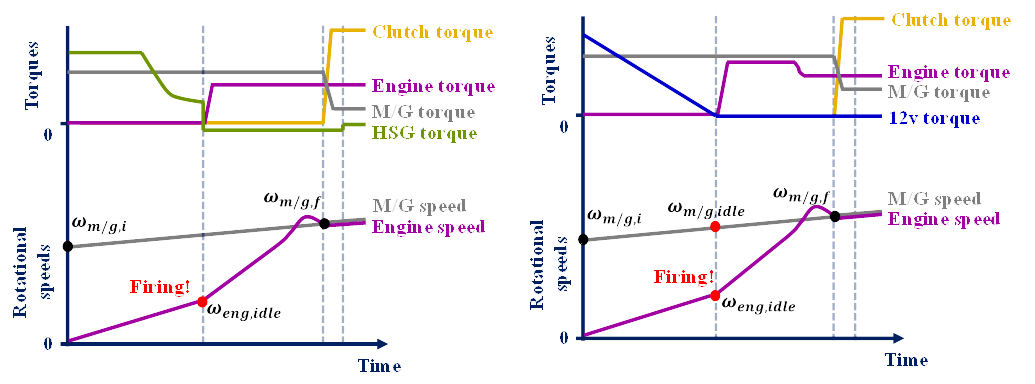
Overview of the P0-P2 engine-start control strategy (좌), Overview of the 12v+P2 engine-start control strategy(우)
- starter motor의 사이즈, 종류와 engine start 성능 사이의 관계를 고려한 구조적 다양성에 대한 연구 진행
- Parallel HEV의 최척 engine start 성능에 대한 체계적인 평가 및 비교 진행