Research
HOME > Research > Research Outputs
Research Outputs
Menu
Data-driven EV Energy Consumption Model
In this work, an EV-specified OBD-II device was self-developed, and it recorded real-world data including driving and charging from multiple commercialized EVs through a participatory research in Jeju island. The total energy in EV system is composed of driving, HVAC, and auxiliary power. Especially, driving energy accounts for over 60% among those demand energies and dominantly determines total energy trend. Therefore, we develop an EV energy consumption model to estimate the driving energy accurately by analyzing motor and battery features from collected real-world data. Moreover, we extend the range of data-driven modeling to a variety of EVs such as EV campers, EV trucks by collecting real-world data.
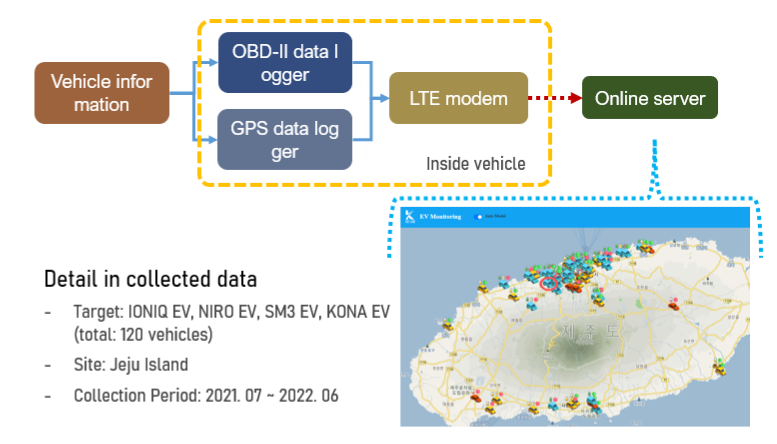
Operation of Micro EV Sharing System
By emerging policies and mobility service for user-active transportation, personal mobility (PM) vehicles have been distributed for first/last-mile mobility service. Micro EV is one of the promising solutions to efficient and eco-friendly transportation for individual mobility. Besides, the flexible mobility property to micro EV is meet with sharing mobility service such as ‘Mobility as a Service (MaaS)’. This study explores optimal routing problem for driving energy, charging infrastructure planning for micro EV operation, and an integrated system which interacts with electric scooters and public transits to overcome the challenging problems and increase the benefits to micro EV.
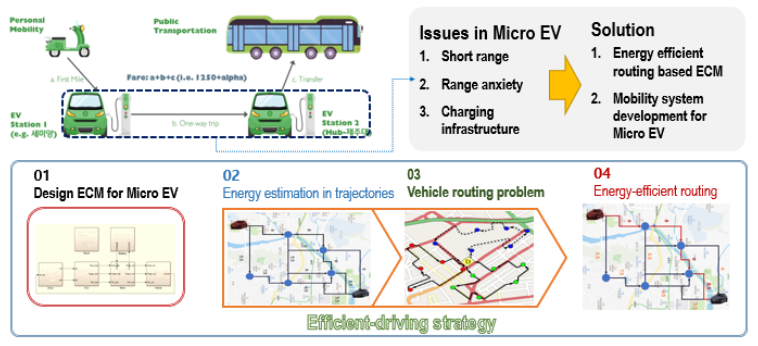
Autonomous Vehicle Operating System for an Unsignalized Intersection
Self-driving cars are equipped with multiple sensors such as GPS, radar, lidar, camera, and IMU to recognize and judge various traffic systems in urban intersection driving environments, but due to limitations in predicting and judging areas outside the sensor’s detection distance, the accident rate is high at 88% of all accidents. Therefore, safe and efficient driving between vehicles through ITS infrastructure-based V2X communication is a key factor in the era of autonomous driving. Considering these points, this study proposes a V2X-based unsignalized intersection autonomous vehicle-to-vehicle control system. For vehicle-to-vehicle control, an Open AI Gym-based simulation environment was established, and a reinforced learning model for information such as location and speed between vehicles was established through DQN and attention mechanism. The network is learned through a cooperative compensation function designed for collision prevention and efficient driving between vehicles, and the result is enhanced learning-based vehicle control, which shows a better effect than conventional unsignalized intersection traffic schemes.
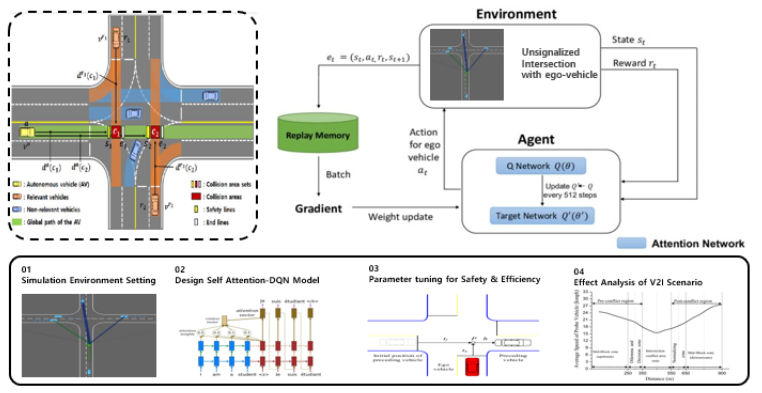